In a previous article, we wrote about the knowledge pyramid and moving through its layers with thought and data analysis to work towards finding actionable insights. In this article, we’d like to run you through another example of this process. We wish to make it clearer by using some demo data and the Feelingstream conversation analytics tool.
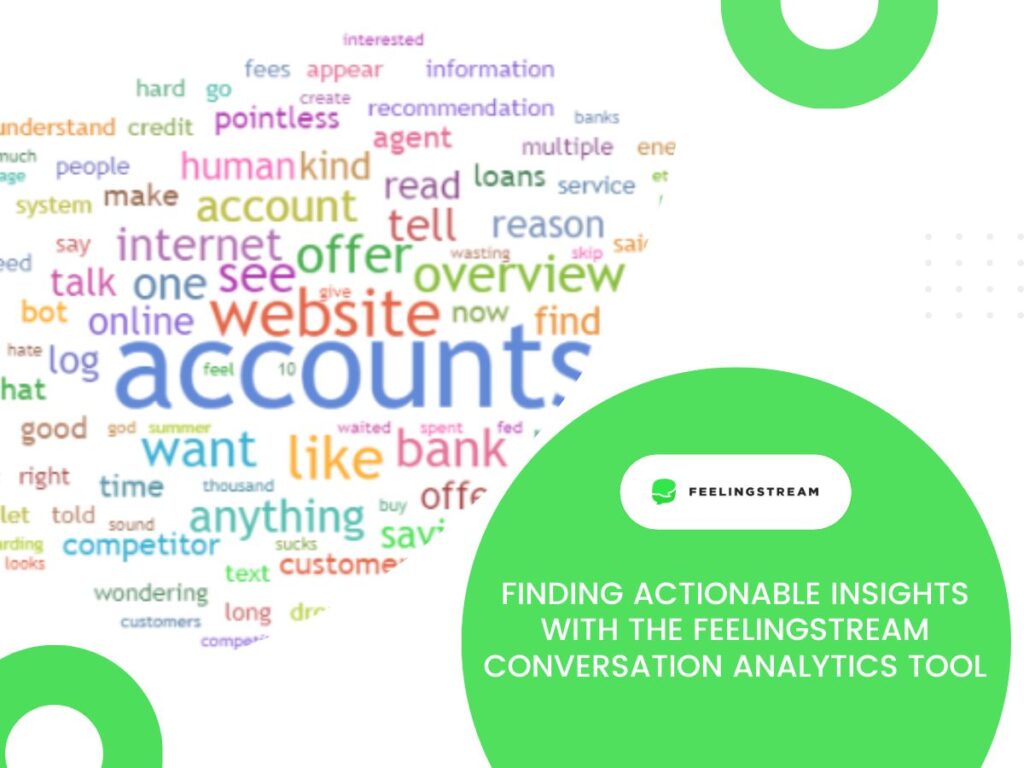
Keep the goal in mind – start with the strategy
When you have collected data from your customer conversations and this is added to the Feelingstream conversation analytics tool, you will find that you can have access to each customer word for textual analysis. You will also be able to investigate conversations via evaluations made on these conversations. It may feel a bit overwhelming and you may not know where to start. Therefore, it’s important that you keep a few things in mind. What are my company’s and my department’s current strategic goals? Related to these goals, what are my business questions?
What are your business questions?
Let’s say your company’s strategic goal is to put more focus on self-service.
Your business questions could be:
“How many contacts do we have regarding our self-service?”
“What are customers finding difficult with our self-service?”
We’re showing these questions or thoughts in this order because investigating customer conversations for working towards your business strategy can be a process done in multiple stages.
Firstly – as we want to improve our self-service, we want to measure the number of contacts we receive regarding self-service. To do that, you could perform quite easy textual searches. You can create charts to look at those conversations over a timeline.
As advised above, we will showcase investigating these business questions on a small sample of chat demo data.
Measuring the issue at hand
To measure the size of this topic, let’s look at the number of conversations that we have in total for our time period. We also want to see how often self-service is mentioned in those conversations.

You’ll notice that a large portion of our conversations include self-service. When looking for mentions of self-service, we did not only look for this keyword. We also included some other ways that the customer or Agent could refer to it. Please look at the setup of this search query.

If your website and self-service site are completely separate from each other, you may not wish to include website mentions in your search. For our demo chats they co-exist and affect each other, we’re looking at them together.
What are customers feeling?
Now, next question – what’s the customer sentiment in conversations with self-service mentions?
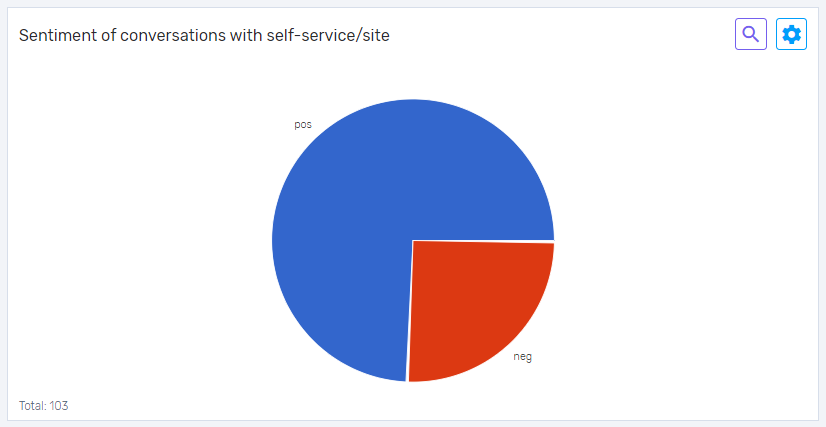
We see that most of the conversations are positive, however, more than 25% are negative. We consider that to be quite high, and it makes us feel like the self-service area needs attention.
In addition to looking at the AI-predicted sentiment of the conversation, we can also look at how many conversations we have where we find indications of issues together with our self-service mentions, an example is below:
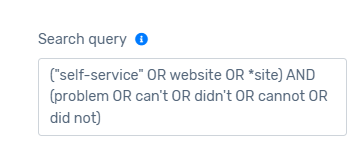
So to answer our first business question, we’d say that self-service or website is mentioned in more than half of our example conversations. More than 25% of those conversations are considered negative. There is definite room for improvement. Let’s move on to further analysis and our next question – “What are customers finding difficult with our self-service?”
Here we’d recommend focusing on analysing all customer conversations that mention self-service and examining the ones with negative sentiment in more detail as well.

In this example above, we’ve put together a Word cloud from negative sentiment conversations that mention self-service and website and are in our demo channel. We’ve also used some cleaning Lexicons to remove unwanted stop words. What we now see, is that “information” is right and center. The focus here is to find unwanted words or hints to customer issues. Instead of reading through transcripts of all our examples (which we could do, if we wanted, of course), let’s try to be focused on words such as “unable” (let’s find what people are unable to do) and “information” (what is this information that they are referring to?).
Take note of the findings, but move on with the strategic goal
Hovering over the Word cloud, we see that the word “unable” comes up 29 times. When we click on that, we see the context in which this word is used.

As you see, our keywords from the search are highlighted and it seems that the word “unable” comes up in a chatbot conversation as part of a phrase the bot says “I am sorry, I am unable to understand what you need assistance with. Please rephrase your query. “. Scrolling through the list of conversations that come up as search results, it seems this phrase has caused majority of the use of the word “unable”. What do we make of this? In the negative conversations, the bot says this phrase at least once – in our example, word “unable” comes up 29 times in 21 conversations.
As our current goal is to find self-service improvements, not ideas for bot improvements, we’ll move on from here (but here’s an idea how you can also focus on bot improvements – find the areas where the bot gets stuck, this can also be done using our tool).
From insight to finding actionable insights
To try to avoid pulling information from the chatbot phrases, let’s refocus our Word cloud on the words of the customer instead, so we can filter the field to query from to customer text. Doing that switches up the words we see in the Word cloud.

The central word is “accounts”. When clicking on that and looking at the search results, the conversations revolve around customers not being able to find the account overview page which is something that should be very central and easy to access. We also see that there are several customers mentioning that they can find the account overview page, but not all accounts are present.
Now we have insights. We see that the self-service topic causes negative conversations. Quite a large number of those conversations lead to the account overview page. What could we do with this information? How to make it actionable? What helps us make the switch from insights to finding actionable insights? We need to turn to the department managing our self-service and provide this information and any customer feedback we have regarding this area of our self-service. This will be valuable in understanding the customer pain and making this area of self-service clearer to access and manage.
Once the self-service is redesigned and the changes go from development to the live customer environment, we can use the Feelingstream conversation analytics tool to monitor for changes – do we see those calls reducing? What do the customers talk about then when asking questions about self-service? Taking this information and customer feedback in one step at a time with monitoring the effects after making changes will give us the option to make gradual changes and reduce our calls over time.
Change is a gradual process
When working towards finding actionable insights, it’s important to start with the strategy and the questions. Also bear in mind that if you find improvement points and insights, you also need resources to take action. Not just physical resources, but people, ownership, time. Finding ways to reduce contacts or improve processes, even if the change seems quite small at the start, is something that starts adding up over time.
If you wish to read more about how conversation analytics could be used in your company, please check out this article here. If you’d want to ask us a question, let us know and email us. We’ll be happy to talk further!