For most businesses, it is very difficult to understand what their customers think of them. They may not know exactly what the customer issues are. Finding data for business decisions is therefore complicated. Managers make decisions either in the dark or based on gut feelings. For businesses that have daily customer interactions and customer service channels, decision making is easier when using conversation analytics.
In this article, we want to share some background to conversation analytics, how we use it in Feelingstream and how our customers can benefit from it.
What is conversation analytics?
Conversational analytics is a process in which usable insights are extracted from human speech and conversation. This is done using natural language processing (NLP) which is a combination of linguistics, computer science, and artificial intelligence.
Businesses can use conversational analytics to evaluate their customer conversations. It can be used to understand voice data from phone calls to gain insight into your customer conversations. Conversation analytics can also be used to study other text-based interactions such as live chat and email.
How does conversation analytics work?
For us at Feelingstream to be able to use conversation analytics, a few things need to happen first.
Speech to text
Firstly, we work on speech analytics. Our customers record their phone calls (ideally there are separate recordings of the speakers – agent and customer). Then we transcribe those voice recordings to text using our own speech to text model. We have created models that we train with audio, transcriptions, and that work for specific languages.
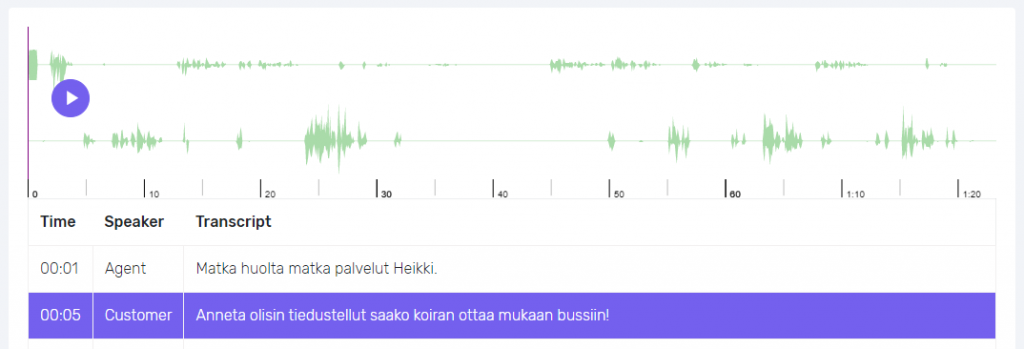
Our focus is spontaneous speech in phone calls, therefore that is also the type of data that we want to make our model the best in.
Textual data
The next step is text analytics or conversational analytics based on analyzing texts. When you have hundreds or thousands of phone calls, chats, or emails available to you, a lot can be achieved with simple keyword searches. With Feelingstream you can review this textual data over a selected timeline. You can filter the texts (only looking at one channel or language at a time, for example) and aggregate the data based on your needs.
Semantic similarity
Users can find keyword searches especially useful if they are combined with semantic similarity. We have developed semantic similarity models for our system to help our users perform keyword searches. These are customer and language specific.
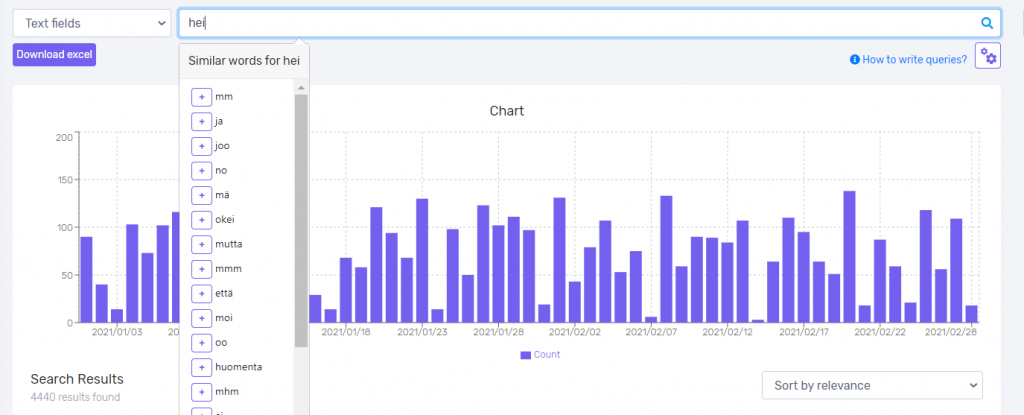
When a user starts entering keywords into the search query field, they have the option of adding additional words that our system offers them based on semantic similarity.
Trying and testing out different keywords and combinations aids in performing searches. The system counts words to give information about specifics – how much a customer mentions a competitor, a service, uses some specific words, etc.
Classification – model creation and use
Classification is another helpful tool that we can use in order to make call tracking, analyzing texts and data easier. Data scientists can use classification for creating various models. Models can assign topics, mark negative conversations, check scripting, etc.
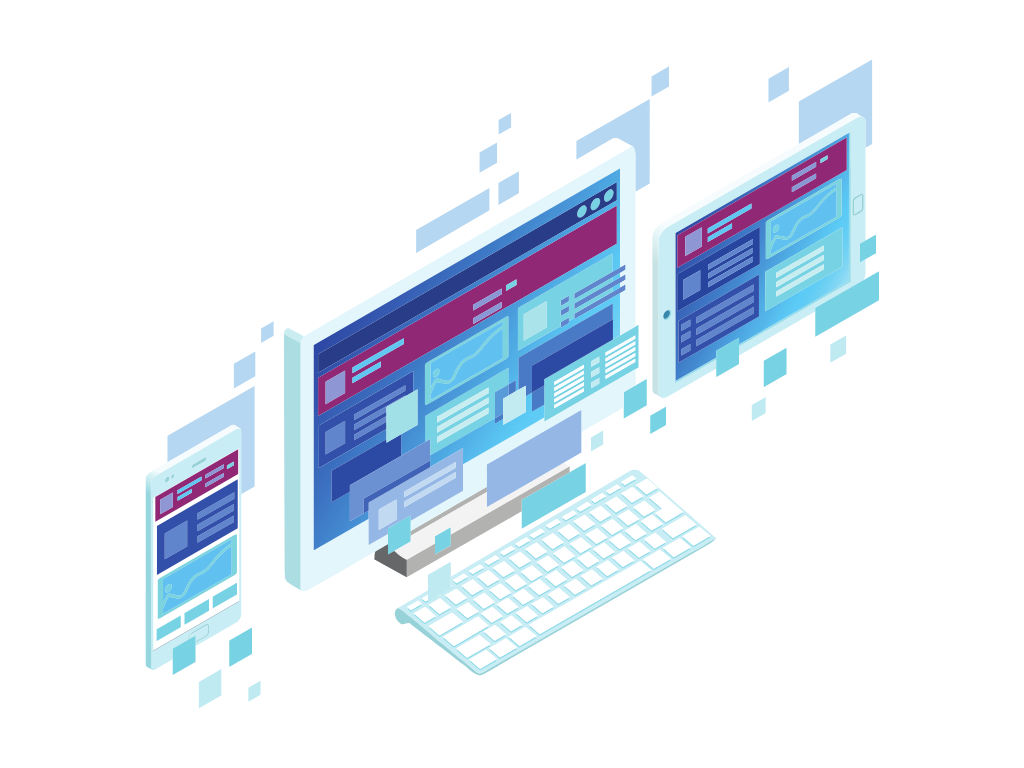
It basically works like this – we collect examples for each category and label the examples manually. Next, we train a model. For a topic model, it learns our examples and starts associating certain words with certain topics.
When the topic model is ready, it can assign categories to new examples. The system can then add automated topics to all conversations. This automated solution is more exact than regular manual topic assigning, it also saves agents time.
It is possible to do unsupervised classification (LDA, for example) where the model learns to associate certain words with topics. The difference is that data scientist does not help the system learn, it creates the topics and categorization itself. Unsupervised classification is not as exact and can offer unexpected results.
Sentiment analysis, sales potential, and churn
We have also created other classifiers such as sentiment – it helps users understand whether the conversation is positive/neutral or negative. Managers can view the agent sentiment and the customer sentiment separately or as a combination of both. Finding calls or conversations with negative sentiment can be beneficial for finding improvement points for processes and services and for agent training.
Other classifiers that we have worked on thus far include sales potential and churn risk. The sales potential classifier can rank calls that have some sales potential. Sales managers can use this information to plan further actions.
The churn risk classifier brings attention to conversations where customers have used vocabulary associated to churn risk and leaving the company. Keeping existing customers is more cost-effective than trying to find new ones. Therefore, managers or agents can take immediate action when there’s churn risk for a customer.
Why does your business need conversation analytics?
Making business decisions can be very difficult. When you have business questions and you have the means to find the data to back your decisions, it becomes much easier. We’ve written an article regarding decision-driven data analysis that we’d recommend for you to read.
Conversation analytics can help businesses become more efficient, for example, reduce time spent on calls by having automatic topic creation and automated after call note processes. Having 100% visibility into customer calls will help quality managers understand the patterns behind the calls that help find information for agent training.
Call quality is another topic that can benefit from conversational analytics. We can use meta-data and data from text and audio for metrics in our analysis. We can measure silence, talk tempo, cross-talking – you name it.
Each company with a large customer service team strives towards better first call resolution and reducing avoidable calls. With conversation analytics, it is possible to reach those goals much easier than trying to gather manual data and act upon that.
What’s next – how could you use conversation analytics?
In conclusion, we hope we’ve given you some clarity into what conversation analytics is and how much you can benefit from it. We in Feelingstream work towards using conversational data in new and innovative ways to give our customers the chance for better business, each day.
If you wish to read more about how exactly you could use Feelingstream to achieve your business goals, please check out other blog posts. We would also be happy to offer you a demo of our services.