Data analysis must always consider privacy needs and regulations. Customer conversations with customer service hold quite a lot of personal data. Therefore, conversation analysis options may be quite limited unless technology is used for data masking or some other method of anonymisation.
Good quality data masking has been a must-have solution for a few of our customers who would not be able to use data analysis with the personal information in there. Personal information does not generally give value for conversation analysis but hinders who access can then be granted to.
In this article, we share four steps to enable entire companies, across various departments and roles, to fully leverage customer conversation analysis through data masking.
Step 1: Named-entity recognition as a starting point for data masking
In natural language processing (NLP), one method of using AI and machine learning is named entity recognition, NER in short. In the context of data masking, NER can be used to find certain pieces of information to be protected and masked, such as names, numbers, addresses, URLs, and emails.
As with machine learning models, there is always a possibility of errors and ambiguities. The model may misinterpret the text and classify a usual noun, as a name for example. Sometimes names and addresses are the same as nouns. For example, someone may be called “Jack Black” whereas “black” is a noun and a name. Therefore, one can never really rely on AI and technology alone here.
Step 2: Validation of results
When a NER model is used to locate personally identifiable information (PII) and mask it, we can assess the quality by doing a thorough analysis of the results. This means humans will have to come to check the results of the technology doing the work – comparing original and masked data. Often, it can be found that the results are quite good, but not always perfect, as the NER models and capabilities are different for different languages. Validation will give us a baseline result and ideas for improvement.
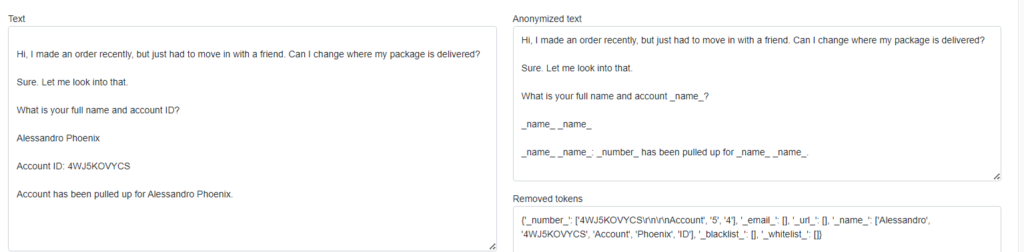
Step 3: Improving the results with blacklisting and whitelisting
Analysis of masked results will show patterns of where the AI system needs improvements. This can be done with blacklisting and whitelisting text.
In this context, blacklisting would mean creating an additional list of words or phrases to be masked in the text. Whitelisting will be used if something is recognised to be masked by the technology, but we want to use it in the conversation analysis process. For example, this may be the case for competitor names, product names.
This process of blacklisting, whitelisting, and validating the results may need to be done a few times to get the best result with data masking. It is all about finding a balance between what must be removed without taking away too much.
With the combination of NER and consistent blacklisting and whitelisting, the data masking accuracy can reach close to 100%.
Step 4: Data masking and privacy for phone call audio
For analysis of phone calls, it may be possible that the user of the conversation analysis may also want to listen to the phone calls. Systematic data masking based on phone call transcripts helps timestamp each masked piece of information which can then be used to mask the audio too. The places where the information must be masked can be whitenoised.
An additional layer of anonymisation can be added by changing the tonality of the audio. This would mean that a person listening to the call would not recognise someone’s voice as the pitch would be changed to be higher or lower than it really is.
Reaping the benefits of data masking in conversation analysis
When privacy concerns and regulations to protect the privacy of customers or customer support agents may be keeping companies back from using conversation analysis, data masking and anonymisation is key.
Check out this article if you wish to find out how the Feelingstream customer conversation analytics tool can enhance your business, harnessing the data masking described above.
Make sure to also read more about how data security can help get most of conversation analysis.
Do you think this solution could be great for your company? Book a demo call here!