Feelingstream’s speech-to-text AI model automatically transcribes customer calls into text. Transcripts of these calls then become searchable for customer insight much like in a search engine such as Google. The Feelingstream’s conversation analytics tool gives our customers the chance to see how customers speak in different customer service channels.
There’s a key difference between searching for information on Google and searching for business critical insight from transcripts of actual calls.
In this post we explain how customer calls are a new kind of data which requires a different way of thinking to find business critical insight.
The difference between making searches on Google and Feelingstream
Searching on Google leads to search results such as texts (and other media) written by people who have a specific message to share. Typically, writers put a lot of thought into how they should write texts to be easily found on Google.
In contrast, Feelingstream’s call transcripts are based on spontaneous talk between two people trying to make sense of each other over the phone moment-by-moment. Speakers focus on the situation they are in. They do not think about how to talk to make their interaction searchable by someone else later on.
In other words, searching for insight from Feelingstream requires a whole new mindset around choosing search keywords.
Searching on Google has become a relatively normal part of people’s lives. Because of that, the first thing that new Feelingstream users often do is to make searches on Feelingstream as if it were Google.
New users typically pick a keyword that relates to their area of work. They then search for calls related to this work. What they often discover is that they fail to find any relevant calls. That is because of the fundamental difference between everyday spontaneous talk and written texts as they appear on Google.
So how exactly is everyday spontaneous talk different from written texts?
Characteristics of spontaneous everyday conversations
For one, people rarely formulate fully coherent sentences when they speak in everyday situations.
What is more common is that people start to speak and not necessarily from the most logical starting point.
Speakers can also abandon their thought to add another thought half-way through. They often make long pauses and use meaningless filler words to their talk.
What’s more, two people having a conversation can sometimes even talk over each other or misinterpret one another. This can potentially lead to a communication breakdown.
With all of the above in mind, our clients are often surprised when they first see transcripts of calls between customers and agents. They unknowingly expect transcripts of calls to look like perfect theatre scripts.
But that’s not how normal people speak in everyday life.
New Feelingstream users are quickly faced with the reality that calls between customers and agents seem long-winded, incoherent and difficult to interpret – at least at first glance!
Indeed, looking for insight from transcripts of spontaneous talk requires new users to switch from thinking in terms of Google keywords to “how would a normal person express this in everyday words”.
Let us share some real life examples, kindly provided by our clients.
Examples of customers wanting to terminate contracts via chat, email and phone – how do the customers speak in different customer service channels?
Feelingstream collects customer interactions from multiple customer service channels into one place. Therefore, we can use real customer interactions (which we have anonymized) to see how spontaneous talk is different from written interactions.
Let’s say we’re interested in customer contacts about customers wanting to terminate their contract.
On Google, we would simply type “terminating service contracts” and get a bunch of results that include this keyword.
However, when we turn to real life situations, customers can express this same goal in very different ways. We’ve seen that their wording depends on their chosen customer service channel.
Below is an example of how a customer would express wanting to terminate a contract through the live chat feature:
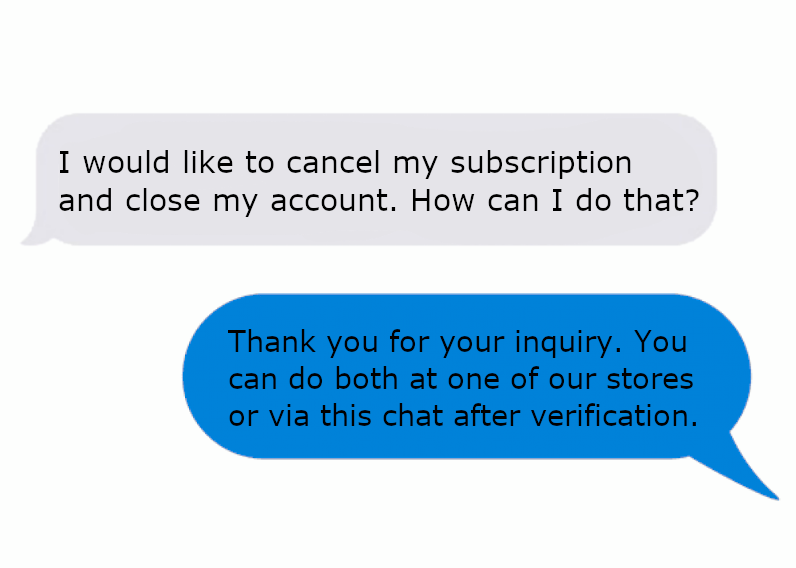
In this live chat interaction we can see that the customer’s request is short and concise. The agent responds in an equally short manner.
In fact, the interaction seems quite straightforward. This is because both customer and agent have had time to think through what they want to say. The end result is a short and sweet information exchange.
However, how would a customer make a similar request via email?
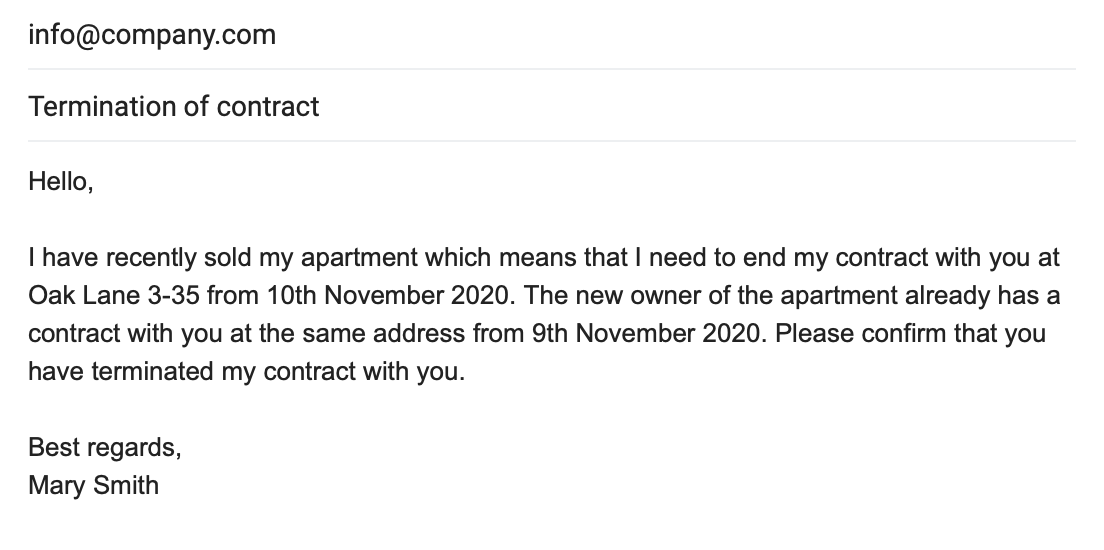
In this customer email we see how the customer lays out the context (selling her apartment) and all the details related to wanting to end her contract.
The customer offers further details regarding her request to terminate the contract. This is to reduce the number of back and forth emails to come with the agent.
Once more, we see that in writing customers have time to think through their request and express it clearly.
However, let us now look at how customers would express their desire to end a contract over the phone.
To illustrate how differently this can be done, we share three examples by three different customers.
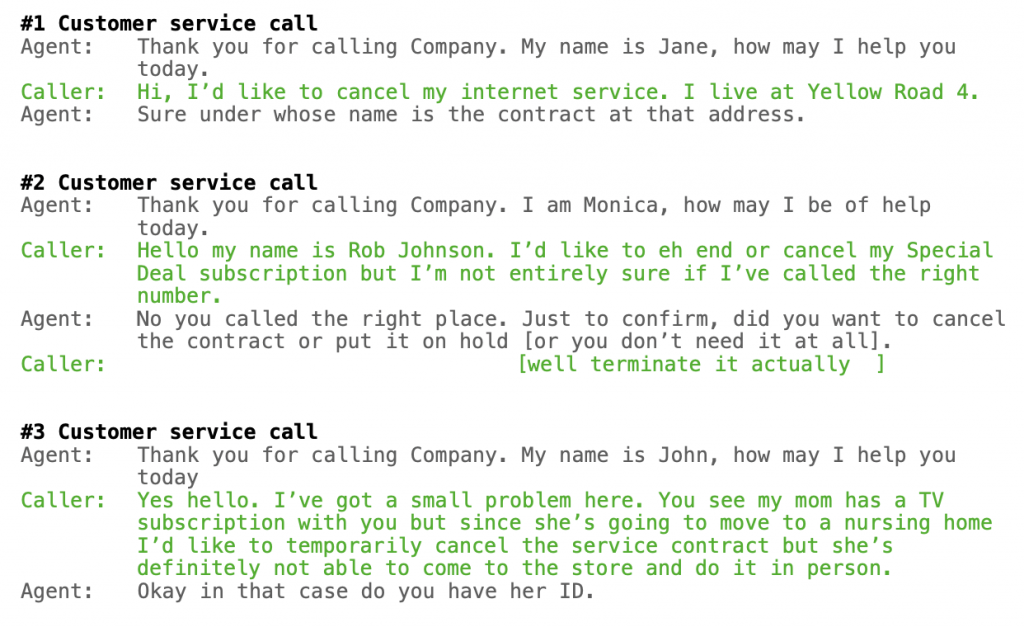
In all three calls we see that the customers express their desire to end their contract or put it on hold in very different ways.
Their reasons for why they want to do so are different. Also the reason why they’re calling to do that differs between calls (rather than using the self-service portal for instance).
When there is such a great variety of ways that customers can express the same thing, what does this mean for finding calls that relate to customers wanting to end their contracts?
The examples above should prove that the search term “terminating service contracts”, which we could successfully use on Google, would not give us very many search results at all.
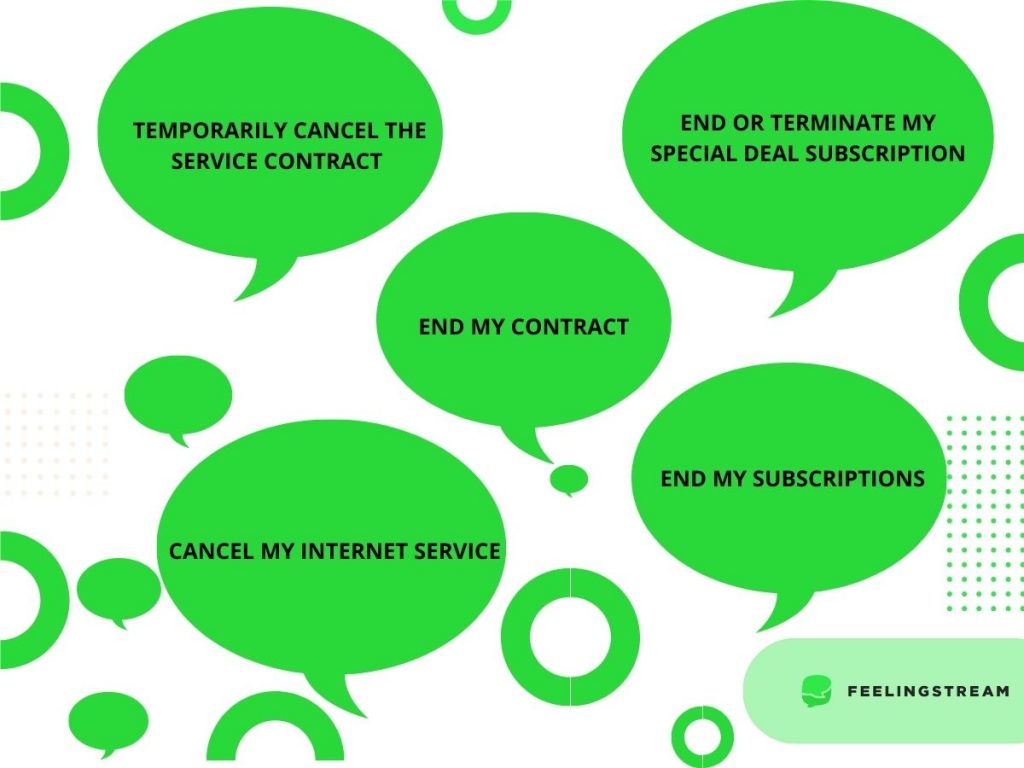
However, when we look through the examples, we discover a number of alternative ways that customers use to express this idea.
Customer interactions are a new kind of data. This requires adopting new ways of finding business critical insight e.g. why are customers leaving. With that in mind, it is these everyday, spontaneous expressions are the real keywords. These are the ones that users should look for on Feelingstream to find relevant calls.
Feelingstream’s speech-to-text and call analytics solution explained – offering a combination of customer service channels to analyse
- Transcripts of calls available after 30 minutes*
- Ad hoc customer insight search supported by AI detection of common expressions and words in customer contacts to simplify the search
- Using just 5 minutes to set up a search gives a statistical overview. See what goes in all calls, emails, or chats – or combine them all. Look at the data over a specified period of time or in a specific team of agents
- We help set up custom searches for monthly customer insight reports, call quality assurance, following sales scripts etc.
If you would like to see a demo of our call analytics feature, get in touch with us.
*Depends on server capacity to save and process audio files.